BCI (Brain-Computer Interface)
Brain-computer interface (BCI) is a communication link between a human’s brain and an external device. EEG measurements can be used in many different BCI systems with some of the examples listed below.
Computer control. Computer or other device control replacing physical input devices such as mouse and keyboard. While BCIs still suffer from being slow, they are invaluable for people who have lost motor function and cannot use physical input devices.
Mind-controlled computer games. While the traditional approach is to emulate the control of a keyboard or joystick, BCI could be used to track an individual’s reaction to various objects, plot twists, and others. This could potentially be used to change the game plot in real time to make it more immersive for a particular individual.
Mental state evaluation. BCI can be used to estimate one’s tiredness, focus, and relaxation level. This is important for people working on critical tasks, for people trying to improve their productivity at work or improve, say, their meditation experience. The estimated tiredness or focus level can be relayed back to the individual in some form of feedback that would improve their focus.
Sleep monitoring. EEG measurements can accurately determine sleep stages and hence can be used for providing insights into sleep quality, waking up a person at the most appropriate time, and others.
Motor function replacement. BCIs can be developed for the control of bionic limbs with the mind. Motor commands coming from the brain can be measured with EEG, which can in turn control a mechatronic limb.
Rehabilitation. It has been recently shown that BCIs can be used to improve the recovery speed of lost motor function by patients who had a stroke. Patients are provided with positive feedback when their brain generates required motor commands by either showing the limb movement on the avatar on the screen or by electrically stimulating the muscles of the limb.
“Lie Detector”. EEG-based BCI can be used to determine if a person is concealing some information. By measuring an individual’s response when various objects are shown it can be determined if a person has seen these objects before or not. It is an ultimate “lie detector” as this brain response is not consciously controlled.
Different BCI paradigms exist within the applications of this technology. These paradigms are related to how our brains function and their response to external stimuli and/or internal mental effort. The most widely used BCI paradigms are summarized in the following sections.
P300
P300 is a type of visually evoked potential that can be observed in EEG recording after a person is presented with visual stimuli. If a person is interested in a particular object, their response is stronger in terms of P300 when such an object is flashed on the screen when compared to other presented objects not relevant to a person.
An example of P300 potential is shown in the figure below. The potential typically occurs approximately 300-400 ms after the presentation of the stimulus, hence the P300 name. Topographically, the strongest response is measured over the parietal cerebral cortex. The ‘target’/’non-target’ waveform corresponds to the person’s response when a stimulus of interest/non-interest is presented.
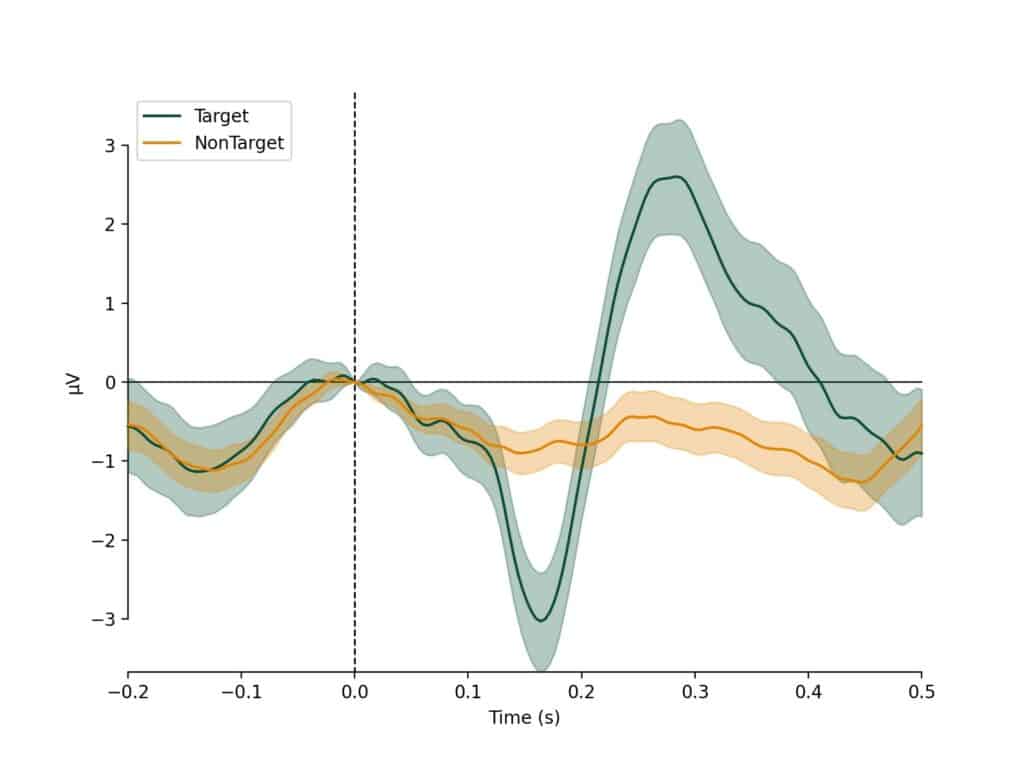
The P300 paradigm can be used for applications where a person can select from multiple presented options simply by focusing on a particular option. A keyboard interface can allow a person to type letters and is particularly useful for people diagnosed with ALS syndrome who can’t use conventional keyboards.
The P300 paradigm can also be employed in a more passive way and, for example, study how a person reacts to various stimuli when using simulators, driving, or operating critical machinery. In the future, this can potentially be used to stop the car or machinery much quicker in critical situations without the need for a person to physically press some pedal or button. The versatility of P300 even extends to the application of criminology, where a suspect can be presented with object photos from a crime scene, and from a P300 response, it can be determined if a person was at the crime scene and saw these particular objects or not.
The peak of the P300 is relatively small and is typically hidden in the noisy EEG recordings and usually, an average of repeated measurements has to be taken to reveal P300 potential as shown in the previous figure.
Here, at Neurotechnology, we have trained machine learning algorithms to detect P300 signals even when they are buried in the noise and would not be detectable by conventional algorithms. We also aim to minimize the number of required electrodes for the detection of P300 potential to minimize and reduce the costs of the required hardware.
It is worth noting that for active control when a person is trying to choose from multiple presented options, the process can be very long when the number of options is large. For example, it can take up to tens of seconds when choosing a letter from a keyboard. Therefore, a very important research direction is trying to minimize this time with the so-called ‘fast P300’ algorithms where stimuli at smaller intervals than 300-400 ms and algorithms have to cope with overlapping P300 potentials in the recordings.
SSVEP
A steady state visually evoked potential is another type of visually evoked potential. Unlike in the P300 paradigm, a user is presented with stimuli flashing at constant frequencies. If a person visually focuses on an object flashing at a particular frequency, the signal of that frequency will be observed in the EEG recordings. Topographically, SSVEP signals are most pronounced over occipital cortex regions. An example of EEG signals with SSVEP signals present in them is shown in the Figure below.
![Spectra of EEG signals when a person is concentrating their visual focus on flickering stimuli of different frequencies]](https://www.brainaccess.ai/wp-content/uploads/2024/02/Spectra-of-EEG-signals-when-a-person-is-concentrating-their-visual-focus-on-flickering-stimuli-of-different-frequencies.jpg)
As with the P300 paradigm, SSVEP can be used in applications where the user can select from various options, such as letters on the keyboard, by simply focusing on a desired option.
SSVEP-generated EEG signals are generally relatively strong and easier to detect than P300 and BCIs based on SSVEP can typically work faster as all the stimuli can be presented at once. However, when the number of flashing options increases, the frequency differences between different stimuli have to decrease in order to fit them in the same frequency range. This can require longer recordings to have a frequency resolution good enough for separating and determining the stimuli that a person is looking at. There are approaches where instead of constant flashing frequencies, coded sequences are used to have more options given the same frequency bandwidth.
Another practical disadvantage of the SSVEP paradigm is that it is quite visually exhausting for a person to focus on various flashing options on the screen for a prolonged time. Hence, there are many attempts to make a more comfortable BCI technology based on SSVEP. For instance, using higher flashing frequencies so that a person is no longer consciously perceived as being flashed, though these frequencies are still presented in EEG recordings. Another option is using various modulation schemes for the stimuli that are less tiring for the eyes but still easily detectable by the algorithm.
Motor Imagery
Motor Imagery (MI) based BCI technology is based on the fact that neuron activity changes in the motor cortex when someone makes or imagines a movement of their own body. The EEG response can also change when only observing the motion performed by someone else.
One of the features that change in EEG recordings associated with the imagined movement is sensorimotor rhythms or oscillations. They are typically present over the motor cortex when no movement is made/imagined but decrease (de-synchronize) over a particular motor cortex region when a particular movement is made/imagined. For example, the rhythms decrease over the left side of the motor cortex if a person imagines a movement of the right leg/arm and vice versa. With higher electrode densities over the motor cortex, it is possible to separate not only the left/right sides but also if the movement is associated with the leg, arm, or even tongue.
The changes in EEG signals associated with MI are generally more subtle when compared to visually evoked potentials and vary greatly between people. Not only are sophisticated algorithms needed to detect MI commands reliably, but sometimes user training is required to elicit MI-related EEG signals.
The application of motor imagery-based BCI technology ranges from object control in slow-paced computer games to allowing disabled people to control exoskeletons. It is also used in neuro-rehabilitation to help people recover lost motor functions more quickly.
Brainwaves
Brainwaves are electrical oscillations or rhythms observed in electroencephalography recordings. Various oscillations can reflect the state of mind of the person such as tiredness, relaxation, concentration, etc. There are associated oscillations with different sleep stages so it can be used to study and determine these.
The BCIs based on the brainwaves are probably the most widely used as the associated EEG signals are relatively strong and typically do not require a large number of sensors and expensive hardware.
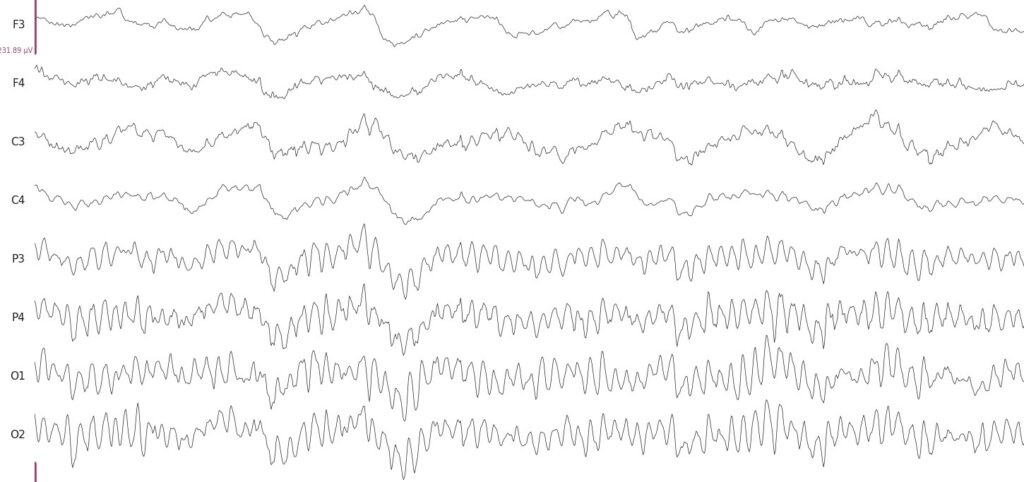
The challenge when using brainwaves for the development of BCI is to find the appropriate feature of the brainwaves that best relates to the state of mind that one wants to detect or classify. In addition to this, elaborate signal processing is needed to minimize the influence of EEG artifacts on the brainwave level measurements.
Challenges of BCI Technologies
BCI technology is undoubtedly a very dynamic and rapidly evolving field. Nonetheless, there are still many technological and practical aspects that have to be overcome in order to see a widespread use of BCI technologies:
Comfortable EEG headwear. Comfortable and portable hardware is essential for applications outside laboratory environments. Not only are dry electrodes necessary but also the actual headwear has to be comfortable enough for the people to wear for a reasonable length of time. If it is used to monitor sleep, for instance, then the headwear and the electrodes have to be very light and comfortable.
Speed. For BCIs to replace conventional computer or other device controls, the control speed should increase considerably. At this stage, they are comparatively very slow, and maybe even new paradigms have to be created to speed them up.
Transferable between sessions. The EEG signals can vary greatly between sessions when the headwear is removed and put on again or different hardware is used altogether. This is due to variations in electrode fitting, their position, environmental conditions such as electrical noise sources, etc. The algorithms have to be able to cope with these changes.
Transferable between people. Not only do the algorithms of BCI technologies have to deal with variation between sessions, but they have to work reliably for different people as well. As every person is generally different there are differences in neural activity too.
EEG datasets. As electroencephalography signals are very complex and as already mentioned vary between sessions and people, we believe it is necessary to use machine learning to achieve better performance than conventional algorithms. Machine learning, particularly deep learning, has shown unprecedented performance in other tasks such as biometry and computer vision and we at Neurotechnology have first-hand experience with this. Nonetheless, machine learning algorithms are ‘data hungry’ and many examples are needed for the algorithm to learn. Currently, there is still a relatively small amount of publicly available EEG datasets.
Please continue reading the next tutorial on hyperscanning, a totally different paradigm for brain activity measurements.